The Downside of Upgrading
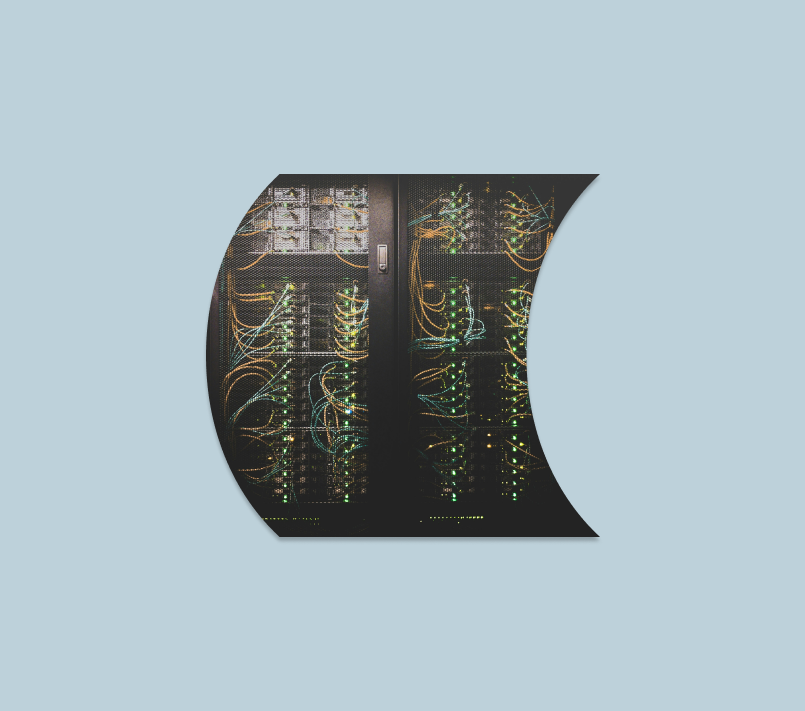
First come the chip factories, then comes the energy suck. We’re not prepared for the environmental implications of fully scaled AI.
By BRIAN CALVERT
Sam Altman desperately needs some semiconductors. He’s concerned he simply doesn’t have enough – the Taiwanese chipmaker NVIDIA currently has a 95 percent market share on these chips – and if he wants to grow his company, OpenAI, he’s going to need billions from investors to start building chips and semiconductors across a global network of fabrication plants. And he’s aiming to ensure there are enough chips to satisfy AI demand by the end of the decade.
We are in a limited window, in other words, to consider the implications of a world where artificial intelligence is fully scaled. Unfortunately, this rapidly closing window coincides with a second closing window, where humanity is racing against time to stop producing greenhouse gases before the planet becomes unlivable.
That means we have about six years to wrangle tough questions about how big AI should get. Where to start?
The environmental impact of artificial intelligence is driven by the energy consumption of its supporting infrastructure, including data centers, cloud networks, and the devices that interface AI with the real world. AI relies on two innovations: neural networks and large language models. Each has significant energy demands.
AI power draw comes from both a training phase, when the machines learn, and the inference phase, when they perform tasks. The more people use AI, the greater the power demand will be from both phases, so as it becomes more widespread, so too do the challenges.
The training of a single deep-learning model can emit as much as 284,000 kg of CO2, the equivalent to the lifetime energy consumption of five cars, according to Dr. Mark Van Rijmenam, a futurist specializing in digital disruption. The carbon emissions associated with AI are on par with those of the airline industry, he writes. And the energy consumption of AI is projected to increase by up to 300 percent by 2025.
Alex de Vries, the founder of Digiconomist, has been studying the unintended environmental consequences of digital trends for the past 10 years. He began by watchdogging bitcoin mining and cryptocurrency but has turned his attention to AI since its explosion in November 2022, when OpenAI introduced ChatGPT to the public.
AI has him more concerned than cryptocurrency because its energy requirements are different: Where crypto-energy is constrained by its niche, AI can be plugged into almost any industry. It will continue to grow exponentially. The bigger the model—the building blocks of machine learning—the smarter the AI. “If you make a model bigger,” says de Vries, “you also need more computational resources and energy to run it.”
That means that even as hardware becomes more efficient, the gains can be overrun by the compulsion to make AI bigger. Models run through chips that are housed on servers that are racked in rows and kept in data centers in the physical world. When an AI program activates, the servers draw power, heat up, and need cooling. An estimated 40 percent of the power draw for a data center goes toward cooling. One large data center can use up to 5 million gallons of water daily. That’s a town’s worth of water (or close to 17,000 households), much of it evaporated and thus unusable even in other liquid applications. And that’s not even a super-heated AI center. We don’t have the numbers for those because the Big Tech companies running AI servers aren’t forthcoming with their numbers.
According to an International Energy Agency forecast published in January 2024, data centers, cryptocurrencies, and artificial intelligence consumed about 460 terawatt hours of electricity worldwide in 2022, almost 2 percent of total global electricity demand. That could more than double by 2026. More than 8,000 data centers are operating globally, a third of which are in the U.S., accounting for 4 percent of U.S. energy demands. Of course, the scale of impact isn’t evenly distributed around the globe; by 2026, the IEA estimates, fully one-third of Ireland’s electricity demand will come from data centers. In Sweden, plans are underway to build a nuclear-powered data center using small modular reactors; it should be up and running by 2030.
On the user end, the agency expects AI to increase the energy load on simple search tools like Google. Where a single Google search currently takes .3 watt-hours of electricity, a ChatGPT request takes 2.9 watt-hours. Were ChatGPT integrated into the 9 billion daily Google searches, that would amount to 10 terawatt hours of additional electricity in a year – enough to power 70,000 homes for a year.
The IEA estimates future energy demand based on forecasts of the number of AI servers estimated to be sold in coming years. NVIDIA currently dominates that market, and in 2023, it shipped 100,000 servers, which in total consume an estimated 7.3 terawatt hours annually. By 2026, based on NVIDIA’s server sales forecasts, the IEA expects the AI industry to grow exponentially, to the tune of ten times its 2023 demand.
As AI continues to develop, e-waste will also increase because hardware life cycles– such as those of server racks, computing equipment, monitors, and circuits – will shorten. A regular server at Meta, for example, has a lifespan of six years, de Vries says. The company can’t even make a prediction for the lifespan of an AI server, though.
If energy constraints don’t restrict the growth of artificial intelligence, it might be hamstrung by the people who use it. There is a lively debate among tech thinkers about whether to “democratize” AI. If artificial intelligence remains centralized with Meta, Google, OpenAI, and other Big Tech companies, the potential for abuse is off the charts.
Imagine a world where AI-enabled mechanisms are always watching us, gathering not just our data but also our images, emails, conversations – all of it. Machine learning has already been used in some courts to prosecute criminals, and it does a terrible job. In 2016, ProPublica published an investigation into the practice with a headline that says it all: “There’s software used across the country to predict future criminals. And it’s biased against Blacks.”
Now, think about how close the world is coming to a fascist resurgence as hard-right parties gain traction amid a backlash to the global economics of the past few decades. Put those together, and you have a nightmare.
The solution could be to get AI into more hands somehow. Currently, only Big Tech has the computing power and resources to run the learning models that teach our machines and run enough computing power to service all the uses of AI. ChatGPT now has 100 million weekly active users, but a democratization push would mean a wider distribution of learning models that are better “taught” so that biases can be addressed and machine intelligence isn’t in the hands of a powerful few.
But once it’s more widely distributed, the technology’s efficiencies wane. Big Tech has a better chance of tackling environmental problems by virtue of its centralization. Redistributing machines and servers – which, as mentioned, run hot and need cooling – puts much more demand on energy grids and water supplies. That means that best practices need to be developed and aligned with good policies so that if AI does decentralize, the environmental damage is mitigated.
Companies large and small can address climate change by reducing their emissions and assisting in mitigation and adaptation measures, argues Anders Nordgren of Linköping University in Sweden. This can be done through energy efficiency and avoiding fossil fuels.
“Given the potential impact of AI on society, it is vital that AI companies and governments take these ethical issues seriously,” he writes.
What can be done to accomplish the goals of mitigating AI’s climate impacts while also democratizing its use? And how soon does it need to be done? Many experts agree that two main areas ought to be a priority: transparency and efficiency. But there are a lot of unknowns to deal with. “If anybody you interview for this story tells you what’s going to happen in five years with the explosion of AI,” says Dennis Wamsted, an energy analyst at the Institute for Energy Economics and Financial Analysis, “I wouldn’t trust him as far as I could throw him.”
Wamsted has been tracking energy and utilities for decades, following a backpacking trip on the Appalachian Trail that taught him the value of efficiency and the strength of experience over theory. (Even in April, he says, prepare for snow.) Right now, we are in the phase of AI where the public and policymakers don’t know what they don’t know – the Rumsfeldian “unknown unknowns.” And the major corporations who do know some of what we don’t know aren’t sharing. AI operates in a black box, making it difficult to make effective policies.
“We need to know how much energy we’re talking about,” Wamsted says, “because you can’t solve a problem if you don’t know what the problem is… We can never know unless we get some real data. And we hardly have any data right now.” So, the first step needs to be policies that push for transparency.
The second policy priority ought to center around efficiency standards, he says. “We have efficiency standards for many things, and there’s no reason we can’t have them for data servers or AI racks,” he notes. Fuel efficiency standards for vehicles pushed innovations for better miles per gallon, and the same could happen for AI. A cap on energy use for a data center, for example, would inevitably lead to energy-lean servers. “So if the increase in electricity use is really, really enormous, there will be a chipmaker or there will be a data center owner that says, ‘You know, there’s a solution here,’” Wamsted says.
Wamsted is also not convinced that AI's growth will be exponential forever. AI may be limited by the simple human factor known as NIMBY-ism. The Not-in-my-Backyard constituency can be ferocious. How many data centers—concrete boxes that offer very few employment opportunities—are too many for one neighborhood? Five? Fifteen? Fifty?
Meta, the parent company of Facebook, recently ran into this issue in de Vries’ home country of the Netherlands. The Dutch already host some 200 data centers, but when Meta moved to open their first one there, the public protested. Meta gave up on the idea after the Dutch senate ordered a temporary halt to construction. “We have a limited supply of renewables,” de Vries says. “We don’t want to be wasting that on a data center that doesn’t even return any jobs to the local community.”
The exponential growth of green power supplies in the US and UK has been curbed for lack of places to put more windmills and solar panels where people won’t complain. Humans enjoy their viewsheds, animals deserve their habitat, and so on. But, Wamsted says, standards that push a data center to cover its roof with solar will mitigate much of the power-demand problem. Some big tech companies have already promised to become We need to be dieting harder and not consuming more calories., and they need to be held to that. “And this is out of my league a little bit, because I’m not the tech guy, if you will,” he says, “but AI could help to solve AI’s energy problem. Right?”
In the worst-case scenario, the energy needs of AI are ignored, even as the world scrambles to curb its greenhouse gas emissions. Right now, humanity has until 2050 to reach net zero on its carbon emissions if it hopes to avoid the worst climate calamities. We’re still way off track on that, by the way. We need to be dieting harder and not consuming more calories. And yet, now we are seeing billions of dollars dumped into AI from China, the U.S., and Europe, potentially accelerating the climate crisis when we ought to be pumping the brakes. If AI grows without the right focus, our goose is cooked.
But there are those who argue that AI, put to proper use, could be a boon to our climate fight. Like Sam Kozel. Kozel is a mountain guy, a snow guy, a ski guy. The kind of guy who would suffer in a warming world of drought, rain, and dwindling snowpack. He earned a master’s degree in environmental management at Western Colorado University in the Rockies and now works remotely out of South Lake Tahoe for a small company, E9 Insight, which gathers energy intelligence for renewable energy advocates.
The world of energy policy is byzantine at best, to the benefit of the major power players. Public utilities – the politically connected, lumbering behemoths of energy production – are overseen by commissions, and any change must come from outside players. In a court, the commissions are the judges, the utilities are the defendants, and anyone looking to change things is essentially a plaintiff. Those plaintiffs – for example, a small group petitioning for a utility to integrate renewables into the grid, or to stop hiking rates for EV charging—may not fully understand all the information that surrounds a utility and may not have the ability to pore over thousands or tens of thousands of pages of public documents, where solutions may be hiding in plain sight.
Instead, AI can ingest these documents, summarize their findings, and provide real intelligence that could lead to real change in the energy sector, Kozel says. “It lets you just access and harness a lot more information a lot more quickly,” he explains. Where once he would need to search a 700-page report for information, he can now teach ChatGPT what to look for. “And very quickly, in one minute or less, I can get a pretty decent and comprehensive summary of anything that touches on what I asked.”
There are other more direct applications of AI, and some very smart people working on them. At Climate Change AI, teams of researchers are searching for ways to address the climate crisis with machine learning. In a seminal paper, researchers associated with CCAI outlined potential climate applications of AI across many sectors: in energy, transportation, buildings and cities, farms and forests, carbon dioxide removal, climate prediction, societal impacts, solar geoengineering, individual action, collective decisions, education, and finance. Learned machines might reduce transportation activity or improve vehicle efficiency, optimize the heating or cooling of buildings, optimize supply chains, or monitor peatlands. They might better forecast extreme weather events, or help a consumer understand their carbon footprint. There seems to be no limit to the imagined capacity of AI.
But what are the trade-offs? Every query Sam Kozel runs consumes time and energy. And as AI is further integrated into our lives, all that time and energy multiplies. We need to figure out whether anything AI gives us can justify this. Is the juice, to borrow a phrase, worth the squeeze? And we need to know now. Because we have no time – or energy – to spare.
Brian Calvert is an environmental journalist based in California. He is the former editor-in-chief of High Country News and has written for The New York Times Magazine, Grist, and Guernica, among others